How to Analyse A/B Test Results Effectively
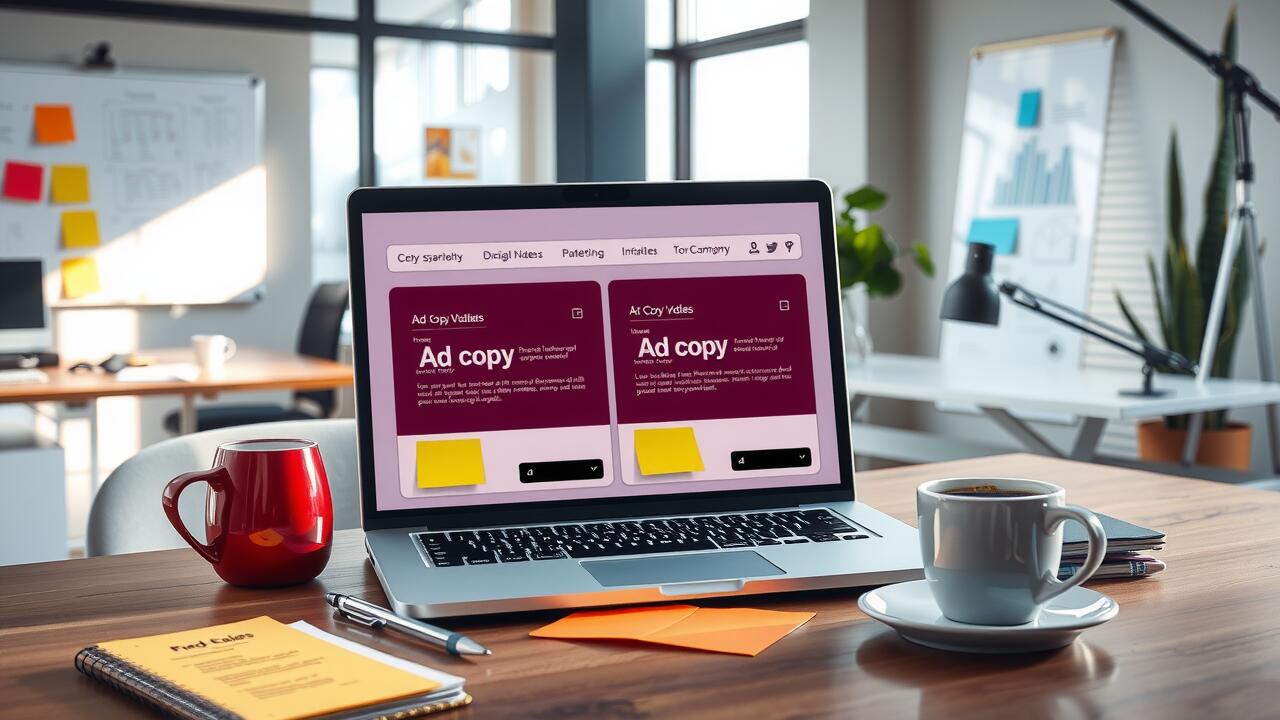
Analyzing A/B Test Results
When analyzing A/B test results, it is important to approach the data with a clear understanding of what metrics are being used. Focus on the specific goals of the test, such as improving conversion rates or reducing bounce rates. Gathering all relevant data is essential for a comprehensive analysis. Utilize data visualization tools to represent the findings in a manner that highlights patterns and trends.
Consider the sample size and duration of the test, as these factors significantly impact the reliability of the results. A larger sample size often leads to more robust conclusions. Furthermore, comparing the performance of the variants while keeping external influences in check can help maintain the validity of the test. By carefully examining each aspect, it becomes easier to draw meaningful insights from the A/B tests.
Statistical Significance Explained
The concept of statistical significance is crucial in evaluating A/B test results. It helps determine whether the observed differences in performance between variations are due to chance or reflect a true effect. Typically, researchers use a p-value threshold, commonly set at 0.05, to assess significance. A p-value below this threshold indicates strong evidence against the null hypothesis, suggesting that the changes made in the test likely led to a real difference in outcomes.
Understanding statistical significance involves recognizing its limitations and potential pitfalls. A test can yield statistically significant results while being practically insignificant in a real-world context. Sample size plays a pivotal role; small samples might produce misleading significance levels. Analysts must consider the size and context of the observed effects, ensuring that actionable insights are drawn with a comprehensive understanding of both statistical and practical significance.
Interpreting the Data
After conducting an A/B test, the next step involves diving into the data collected to extract meaningful insights. Begin by examining the key metrics chosen at the outset of the experiment. These metrics, whether they are conversion rates, click-through rates, or user engagement levels, serve as the foundation of your analysis. Look for patterns in the data that indicate how variations performed against each other. Be cautious about focusing solely on averages, as they can sometimes obscure important details of how different segments of your audience reacted.
Understanding the distribution of your data is equally important. Utilizing tools such as visualizations can help reveal trends and anomalies that numerical summaries alone might miss. Pay attention to the sample size, as smaller samples may lead to misleading interpretations. Employ statistical techniques to explore the significance of your findings. Identifying whether observed differences are statistically significant enhances the reliability of your conclusions and guides future actions for improving user experience or conversion rates.
Understanding Confidence Intervals
Confidence intervals offer a range of values that likely contain the true parameter of interest. These intervals provide a measure of uncertainty surrounding an estimate derived from an A/B test. Typically expressed at a certain confidence level, such as 95%, they indicate that if the same experiment were repeated numerous times, approximately 95% of those intervals would encompass the true average outcome. Understanding this concept helps researchers gauge the reliability of their findings, adding a layer of robustness to the conclusions drawn from the data.
Interpreting confidence intervals correctly is vital for informed decision-making. A narrow interval suggests precise estimates and greater confidence in the results, while a wider interval indicates uncertainty. If the interval overlaps with zero or the null hypothesis, it may imply that the observed difference is not statistically significant. Knowing how to assess these intervals assists in distinguishing between meaningful results and random variations, guiding strategies and next steps in the testing process.
Making Data-Driven Decisions
The insights gained from A/B testing serve as a solid foundation for decision-making. By analyzing user behavior and preferences, businesses can identify the elements that resonate with their audience. This approach allows for adjustments in marketing strategies and product development, ensuring that changes align with consumer needs. Teams gain clarity on which variations lead to better outcomes, facilitating more informed choices based on real data.
Incorporating test results into overarching strategies requires a careful evaluation of the findings. It is essential to prioritize actions that drive growth while supporting the overall business objectives. Regularly revisiting and refining these strategies can enhance performance and engagement over time. By fostering a culture that values data-driven insights, organizations position themselves for sustained success in a dynamic market environment.
Integrating Results into Strategy
Once A/B test results have been carefully analyzed and validated, the next step involves weaving those insights into broader business strategies. This process requires an understanding of how the findings can inform product development, marketing initiatives, and user experience enhancements. Identifying patterns from the data allows teams to pinpoint what resonates most with users, guiding decisions that may lead to improved engagement and conversion rates.
Incorporating A/B test findings into strategy also means fostering a culture of experimentation within the organization. Leaders should encourage teams to view failures as learning opportunities, promoting continuous refinement of campaigns based on data-driven evidence. Regularly revisiting test results provides fresh insight and helps teams stay aligned with user preferences, ultimately leading to more robust and adaptable business strategies.
FAQS
What is an A/B test?
An A/B test is a method of comparing two versions of a webpage or app against each other to determine which one performs better in terms of user engagement, conversion rates, or other key metrics.
How do I know if my A/B test results are statistically significant?
Statistical significance can be determined using p-values or confidence intervals. A common threshold for significance is a p-value of less than 0.05, indicating that the observed differences are unlikely to occur due to random chance.
What is a confidence interval, and why is it important in A/B testing?
A confidence interval is a range of values that likely contains the true effect size. It provides insight into the precision of your estimates and helps determine whether the results of your test are reliable and actionable.
How can I interpret the data from my A/B test effectively?
To interpret the data effectively, compare the performance metrics of both versions, assess statistical significance, and consider external factors that may have influenced the results. Look for patterns and insights that can inform your decision-making.
How should I integrate A/B test results into my overall strategy?
A/B test results should be used to inform business decisions and marketing strategies. Analyze the insights gained, adjust your approach based on successful outcomes, and continuously test new hypotheses to optimize performance further.
Related Links
A/B Testing in Ad Copy DevelopmentHow to Conduct A/B Testing for Google Ads
Roundup of Best Practices in A/B Testing for Ads