Mastering A/B Testing and Experimentation for Effective B Testing Strategies
Table Of Contents
Key Takeaways
- Summary of A/B Testing Concepts
- Variations of A/B Experiments
- Successful Strategies for A/B Testing
- Common Tools for A/B Experiments
- Recommended Techniques for Experimentation
- Analyzing A/B Testing Outcomes
Overview of A/B Testing
A/B Testing and Experimentation serve as powerful methodologies in data-driven decision-making processes. A/B testing allows businesses to compare two versions of a webpage, app, or marketing message against each other to determine which performs better. By conducting various a/b tests, organizations can gain valuable insights that inform future strategies. Experimentation becomes a critical driver of growth, enabling teams to understand user preferences and behaviors through rigorous testing. The ability to formulate testable hypotheses and analyze results ensures that each experiment contributes to more effective outcomes. With a focus on A/B Testing and Experimentation, companies can continuously refine their approaches based on empirical evidence.
Test Version | Metric Being Tested | Audience Segment | Conversion Rate (%) | Notes |
---|---|---|---|---|
Control (A) | Call-to-Action Button Color | New Visitors | 3.2% | Standard blue button |
Variant (B) | Call-to-Action Button Color | New Visitors | 4.5% | Orange button tested |
Control (A) | Email Subject Line | Existing Customers | 15% | Standard subject line used |
Variant (B) | Email Subject Line | Existing Customers | 18% | Urgent subject line tested |
What is A/B Testing?
A/B Testing involves comparing two or more variations of a website or product to determine which one performs better. This process is a crucial aspect of experimentation and typically employs controlled experiments to test specific hypotheses. The use of statistical methods such as the t-test or g-test helps in analyzing the results. By carefully formulating hypothesis tests, researchers can derive insights on user behavior and preferences, allowing them to make data-driven decisions.
The practice of A/B Testing and Experimentation not only aids in understanding user interaction but also enhances conversion rates through systematic testing statistics. Sequential testing can provide real-time insights while minimizing the risk of errors in decision-making. By rigorously analyzing results using appropriate hypothesis tests, marketers and product developers are empowered to optimize their offerings effectively.
Importance of Experimentation in A/B Testing
A/B Testing and Experimentation are essential strategies for understanding user behavior and optimizing digital experiences. Through proper experiment design, marketers can conduct user testing that leverages statistical hypothesis testing methods, such as z-tests and student's t-tests. These tests enable teams to create testable hypotheses, facilitating the evaluation of various elements, including copy testing and layout adjustments. By employing multivariate testing, businesses can gather deeper insights into how different variables interact and affect conversion rates.
The significance of solid experimentation lies in its ability to extract actionable insights from A/B testing results. Employing binomial tests helps in determining the statistical significance of findings, ensuring that the results are not due to random chance. Understanding the data generated through A/B Testing and Experimentation fosters a culture of informed decision-making. This approach allows organizations to refine their marketing strategies continually, enhancing user engagement and improving overall performance.
Types of A/B Tests
A/B Testing and Experimentation encompass various methodologies to optimize decision-making through data-driven insights. Standard A/B tests typically involve comparing two versions of a webpage or product, where statistical methods such as student's t-tests and two-tailed tests assess the significance of the results. Multivariate tests expand on this concept by evaluating multiple variables simultaneously, allowing for a deeper understanding of user behavior. Split URL testing serves as an alternative by directing users to different URLs, which is particularly useful for larger changes. Employing fisher's exact test in randomized controlled experiments can further enhance the analysis, especially when dealing with small sample sizes. The focus remains on addressing a specific research question and ensuring the integrity of results through rigorous scientific methodology, including usability tests to evaluate user experience comprehensively.
Standard A/B Tests
A/B Testing and Experimentation serve as fundamental tools in the realm of research methods, allowing businesses to compare two versions of a variable to determine which performs better. Utilizing a randomized experiment format, this approach adheres closely to the principles of scientific method and experimental design. Common statistical tests, such as the chi-squared test and Mann–Whitney U test, are often employed to analyze outcomes and draw conclusions from samples.
This type of testing is particularly effective for evaluating web page design, marketing strategies, and product features. It provides insights that guide decision-making, ensuring that changes are grounded in data rather than assumptions. By carefully managing samples and implementing a rigorous research method, A/B Testing and Experimentation can lead to significant improvements in user engagement and conversion rates.
Multivariate Testing and Its Applications
Multivariate testing is a robust approach within A/B testing and experimentation that allows marketers to analyze multiple variables simultaneously. This method goes beyond simple A/B testing practices by enabling businesses to understand how different elements of a webpage interact. By offering A/B testing with various combinations, organizations can optimize their designs and strategies more effectively. Utilizing advanced A/B testing tools makes this process less cumbersome, allowing for quicker insights and improved decision-making.
Applications of multivariate testing are vast and can significantly enhance marketing efforts. Many popular A/B testing tools now incorporate this technology to help businesses refine their conversion rates and user engagement. With effective A/B testing initiatives, companies can identify the most impactful combinations of elements, leading to superior performance. Through careful analysis of results from b testing, marketers can implement data-driven strategies that resonate better with their target audiences.
Split URL Testing
Split URL testing involves directing users to different URLs to compare variations of a webpage or marketing asset. This approach is particularly valuable within an A/B Testing and Experimentation framework, allowing businesses to execute A/B tests that evaluate fundamental changes in layout, design, or content. By leveraging split URL testing, companies can effectively assess the impact of different A/B tests on user behavior and conversion rates.
This method contrasts with typical A/B tests where variations appear on the same URL. Split URL testing offers unique insights through A/B/n tests, which can include multiple variations and enhance the depth of analysis. Proper A/B test execution and split testing help clarify user interactions and preferences. By adopting this strategy, organizations can refine their A/B test engineering and foster a culture of experimentation.
- Split URL testing enables a clear comparison of different page designs or content.
- It can improve conversion rates by identifying the more effective version of a webpage.
- This testing method allows for more fundamental changes that may not be possible with standard A/B tests.
- Organizations can manage multiple variations simultaneously for broader insights.
- Split URL testing can lead to improved user experiences by tailoring content to user preferences.
- It supports data-driven decision-making by providing detailed performance metrics.
- The method encourages a systematic approach to experimentation within marketing strategies.
Effective A/B Testing Strategies
A/B testing is a powerful approach that relies on well-defined strategies to drive successful outcomes. Crafting a robust A/B test design is crucial for achieving valid A/B tests, especially when dealing with traditional A/B tests or more complex experimentation methods. By establishing clear goals before an A/B test starts, teams can effectively measure success and utilize the insights gained. Analyzing past A/B tests enhances understanding of what works, informing future experimentation capabilities. The choice of an appropriate A/B testing platform can streamline the process, making it easier to run multiple A/B tests concurrently while ensuring accurate comparisons. Focusing on these strategies will significantly enhance the effectiveness of any A/B testing and experimentation initiative.
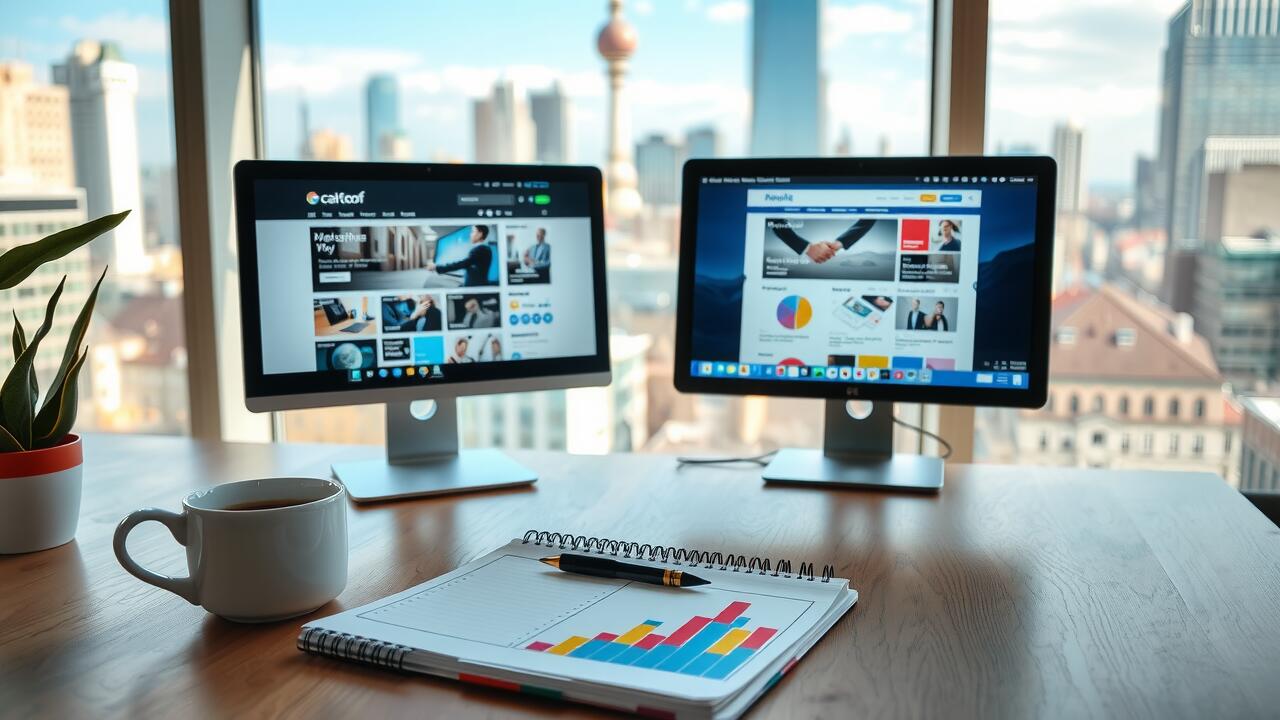
Defining Clear Goals for A/B Tests
Establishing clear goals is crucial for effective A/B Testing and Experimentation. Defining specific objectives helps guide the design of your tests, whether you are conducting classic A/B tests or more complex a/b/n tests. Successful A/B tests stem from well-articulated goals that align with your overall business strategy. This clarity also assists in interpreting A/B test results accurately and ensuring that subsequent A/B tests build on insights gained from previous experimentation.
Clarity in your goals not only enhances the focus of your tests but also aids in selecting appropriate metrics for measuring success. By understanding what you aim to achieve, you can differentiate between a/b tests and a/a tests, tailoring your experimentation strategies to yield the most relevant insights. These insights drive more informed decisions that capitalize on the opportunities experimentation offers, leading to improved performance and optimization in your campaigns.
Choosing the Right Metrics for Success
Selecting the appropriate metrics is crucial for the success of A/B Testing and Experimentation. Metrics provide the framework for understanding the efficacy of your test ideas. Rapid testing helps in facilitating timely decisions, but without clear metrics, it becomes challenging to interpret the results. The experimentation process thrives on clarity, so defining what success looks like beforehand will lead to more reliable outcomes. An A/A test can also be beneficial in establishing a baseline for performance, ensuring that any variations in results from the big A/B test can be attributed to the changes implemented.
Metrics should align with specific goals of the testing initiative. Clear metrics enable informed experimentation strategies that can drive actionable insights. Experimentation encourages businesses to utilize techniques such as controlled experimentation to effectively compare different variations. Each metric serves a purpose in guiding the experimentation play, whether it’s conversion rates, user engagement, or other KPIs. Properly chosen metrics will provide a solid foundation for assessing the impact of your tests, ensuring that you can confidently iterate on your findings and optimize future testing efforts.
Sample Size Calculation and Statistical Power
Determining the right sample size is crucial for the success of any A/B Testing and Experimentation initiative. A properly calculated sample size ensures that testers have enough data to draw meaningful conclusions from a controlled test. Insufficient sample sizes can lead to inconclusive results, while excessive sizes may waste resources. In the context of an a/b/c/d experiment, having a robust sample size allows for an effective comparison of multiple variations. This careful planning enhances the rigor of the testing framework and supports continuous experimentation in the long run.
Statistical power plays a pivotal role in A/B Testing and Experimentation by helping to identify the likelihood of detecting an effect when there is one. A testing framework that incorporates proper power analysis allows teams to make data-driven decisions, reducing the chances of committing Type I and Type II errors. For systematic experimentation, understanding how sample size influences statistical power is vital. The interplay between these elements ensures that every a/b/c/d test is not only scientifically sound but also relevant to stakeholders' goals.
Popular A/B Testing Tools
A/B Testing and Experimentation play a critical role in refining marketing strategies and enhancing user experience. Various platforms are available to support test developers in their efforts to conduct effective experiments, enabling them to create new tests with precision and speed. These tools facilitate the execution of simultaneous tests, allowing teams to explore multiple hypotheses and gather data on their a/b test hypotheses in real time. By fostering a robust testing culture, organizations can leverage insights gained from research experiments to drive their experimentation initiatives forward. High-tempo testing environments create opportunities for rapid iteration, ensuring that teams can continuously adapt based on the outcomes of their experiments.
Tool Name | Key Features | Suitable For |
---|---|---|
Optimizely | Visual editor, multivariate testing, target audience segmentation | Developers and Marketing Teams |
VWO (Visual Website Optimizer) | A/B testing, heatmaps, user feedback tools | Marketers and Product Managers |
Google Optimize | In-browser editor, integration with Google Analytics, targeted audiences | Small to Medium Businesses |
Adobe Target | A/B testing, personalized content delivery, multivariate testing | Enterprise Organizations |
Unbounce | Landing page A/B testing, drag-and-drop builder, conversion tracking | Marketing Teams and Freelancers |
Overview of Leading A/B Testing Platforms
Many businesses rely on A/B Testing and Experimentation to optimize their marketing strategies. Leading experimentation platforms provide the necessary tools to set up multiple tests efficiently. These platforms allow teams to run many experiments simultaneously, ensuring that they can test everything from landing pages to email content. With robust analytics, they can determine how each experiment performs and adjust their strategies accordingly to maximize conversion testing.
Choosing the right platform for A/B Testing and Experimentation is crucial for successful outcomes. A leading experimentation platform should not only facilitate the first test but also support ongoing product experimentation. By leveraging the capabilities of these appropriate testing platforms, teams can quickly analyze results and iterate on their hypotheses, ultimately driving higher conversion rates and informed decision-making throughout their marketing efforts.
Comparing Features of Different A/B Testing Tools
A/B Testing and Experimentation platforms vary significantly in their features, making it crucial for experimenters to understand the strengths of each tool. Many platforms support various test designs, allowing users to create diverse test variations with ease. Features such as drag-and-drop interfaces enhance user experience while simplifying the setup of multiple experiments. Other tools may focus on advanced statistical testing methods, like t-tests, helping users analyze results more reliably after each test finishes.
The capacity to conduct online experimentation efficiently can set a tool apart. Some platforms offer robust analytics that automatically adjust for sample size and statistical power, optimizing test runs. Future experiments benefit from insights gathered from previous tests, ensuring that experimenters can make data-driven decisions. By comparing the features of different A/B Testing and Experimentation tools, organizations can better align their strategies with the goals of their testing efforts.
Best Practices for Experimentation
Successful A/B Testing and Experimentation relies on a structured testing framework that guides the design of controlled experiments. Each designed experiment should incorporate tailored experiments aligned with clear objectives. Utilizing an A/B test calculator ensures that sample sizes are adequate, enabling reliable and statistically sound results. Test time can significantly affect the validity of the experiment results; hence, it is crucial to allow ample duration for meaningful data collection. Iterative testing helps refine hypotheses and improve outcomes, making it easier to interpret test results accurately. By adhering to these best practices, teams can enhance the quality and impact of their A/B Testing and Experimentation efforts.
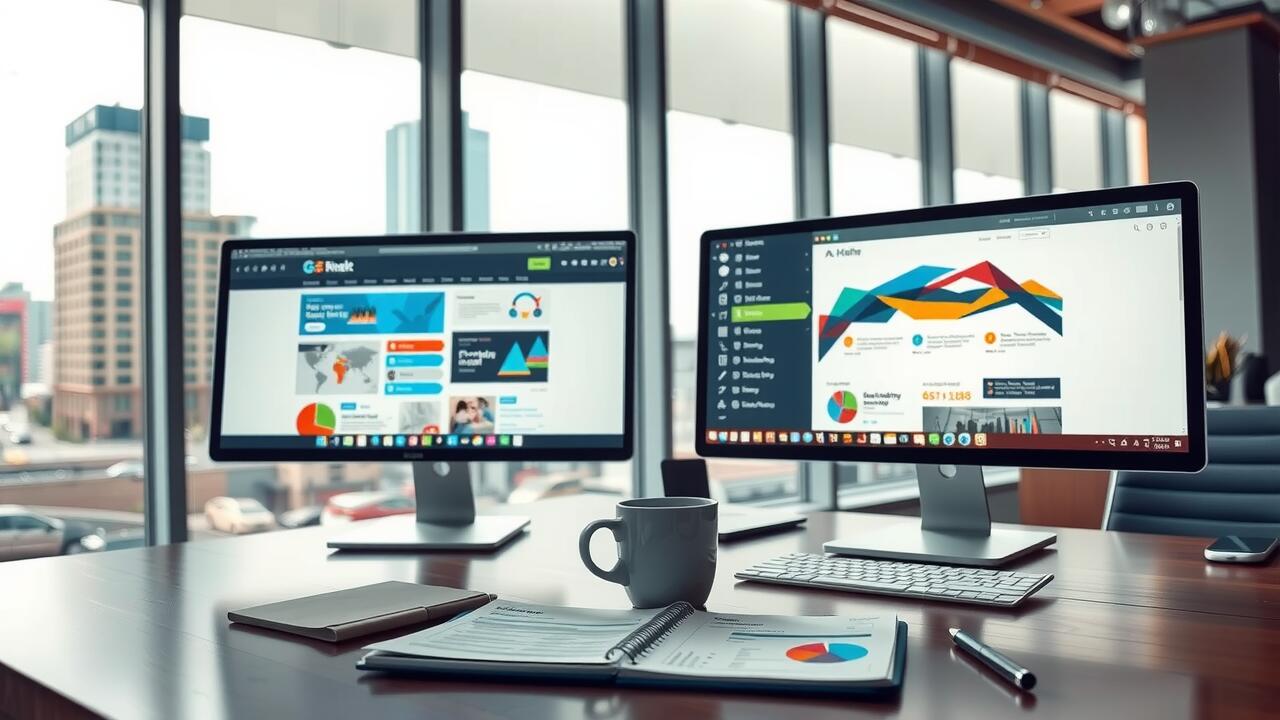
Designing Your A/B Tests for Maximum Impact
Effective A/B Testing and Experimentation hinges on proper planning and design. An impactful experiment begins with selecting the right elements to test, ensuring that both variations are distinct yet aligned with the overall goal of the same experiment. Experimenters must focus on aspects such as headlines, calls to action, or layouts, as these elements significantly influence user behavior. By crafting a clear hypothesis and establishing control parameters, the groundwork for a successful A/B test landing is established, leading to meaningful insights.
Choosing metrics to measure test results is crucial for meaningful outcomes. Effective A/B Testing requires consideration of both quantitative and qualitative data to evaluate the success of the product experimentation method. Common pitfalls include overlooking potential test failures due to insufficient sample sizes or extended test durations, which can skew results. By reassessing past experiments and incorporating learnings, designers can refine their approach, ultimately leading to more effective A/B tests and valuable insights for future experimentation.
Analyzing Past A/B Tests for Insights
Reviewing past A/B Testing and Experimentation results is a crucial step in refining future strategies. This analysis allows experiment assessors to understand which total test versions yielded the best outcomes and which resulted in failed A/B tests. Learning from previous experiments can help in crafting more effective testable statements for future controlled experiments. By focusing on the successes and failures of previous A/B split tests, teams can identify patterns that enhance decision-making processes.
Harnessing insights from complex experiments can significantly improve the efficacy of advanced A/B Testing and Experimentation approaches. Utilizing two-sample hypothesis tests helps pinpoint what worked well and what did not during past tests. By reflecting on the lessons learned from earlier tests, organizations can develop more robust strategies that lead to better-performing campaigns. This iterative process ensures that every experiment builds upon its predecessor, ultimately optimizing the testing framework.
Interpreting A/B Test Results
Understanding the outcomes of A/B Testing and Experimentation requires careful analysis of test data collected from online experiments. Effective interpretation centers on distinguishing between significant and non-significant results, often guided by the principles of a two-sample hypothesis test. This involves assessing how well the challenger experiment performs against the control, with particular attention to potential test variables that may impact user experience testing. Cross-device testing also plays a prominent role in ensuring results are reliable across different platforms. Running tests without a clear understanding of statistical significance can lead to misinterpretation, making it critical to analyze data methodically for actionable insights.
Understanding Statistical Significance
Statistical significance is a crucial aspect of A/B Testing and Experimentation. It helps determine whether the differences observed in many test results are due to actual changes in behavior or simply random variation. A powerful test must be meticulously designed to demonstrate meaningful experiment impact. Understanding how to interpret statistical significance can guide marketers and product teams in making confident decisions based on experiment outcomes, fostering a robust test-and-learn mindset.
Interpreting test outcomes correctly requires knowledge of various statistical principles. Misunderstandings can lead to flawed conclusions that undermine the iterative testing process. A suitable A/B test not only highlights significant differences but also establishes a foundation for future experiments. By grasping the nuances of statistical significance, teams can ensure that their A/B Testing and Experimentation efforts yield actionable insights that drive improvements.
- Statistical significance indicates whether results are likely due to real effects rather than chance.
- A common threshold for statistical significance is a p-value of less than 0.05.
- Larger sample sizes tend to provide more reliable results and enhance the ability to detect significant differences.
- Confidence intervals offer additional context by indicating the range within which the true effect size may lie.
- Effect size is important to evaluate the practical significance of the results beyond just statistical significance.
- Repeated testing helps validate results and reduces the risk of Type I and Type II errors.
- Understanding the context of your data is essential to make informed decisions based on test results.
Common Pitfalls in Analyzing A/B Test Results
A common pitfall in A/B Testing and Experimentation arises from neglecting pre-experiment data, which can provide crucial context for understanding the results. Without this information, teams may misinterpret the performance of the tested element. For example, if copy tests are executed without a clear benchmark, it can lead to misleading conclusions. Organizations must establish their experiment goal clearly and ensure that their testing environment utilizes scalable testing frameworks to maintain accuracy in the results.
Another frequent mistake involves the misuse of client-side testing tools. These tools can facilitate smarter experiments but can also introduce bias if not implemented correctly. Split-run testing, when poorly designed, may lead to miscalculations of binomial tests measure, skewing the data. By ensuring that the parameters of the A/B tests are well-defined, teams can avoid many of the pitfalls that plague analysis, leading to more reliable insights from their experimentation efforts.
Conclusion
A/B Testing and Experimentation serve as essential frameworks for optimizing user experience and enhancing decision-making in various fields. With multiple testable statements in mind, marketers can create controlled experiments to compare total test versions effectively. Employing advanced A/B methodologies, such as complex experiments and split tests, allows for a deeper understanding of audience behavior. An experiment assessor plays a crucial role in identifying insights from both successful and failed A/B tests, ensuring that the findings from previous experiments inform future strategies. Through the application of two-sample hypothesis tests, businesses can achieve statistically significant results, ultimately driving greater performance and growth.
FAQS
What is A/B testing?
A/B testing is a method of comparing two versions of a webpage, app, or other content to determine which one performs better. It involves dividing traffic between the two variants and measuring their performance based on specific metrics.
Why is experimentation important in A/B testing?
Experimentation is crucial in A/B testing because it allows businesses to make data-driven decisions, understand user preferences, and optimize their content or products for improved performance and conversion rates.
What are the different types of A/B tests?
The different types of A/B tests include standard A/B tests, multivariate testing, and split URL testing. Each type serves different purposes and helps in identifying the most effective variations of a webpage or app.
How do I choose the right metrics for my A/B tests?
Choosing the right metrics for A/B tests involves identifying the goals of the test, such as increasing conversion rates, reducing bounce rates, or improving user engagement. Metrics should align with these goals to effectively measure success.
What are common pitfalls to avoid when analyzing A/B test results?
Common pitfalls in analyzing A/B test results include misinterpreting statistical significance, failing to account for external factors, analyzing data too early, and not having a clear hypothesis before starting the test. Avoiding these can lead to more accurate insights.
How can I conduct multiple A/B tests effectively while ensuring proper design and execution in my experimentation program?
To conduct multiple A/B tests effectively, it is essential to have a structured approach that involves designing experiments meticulously and following an A/B testing framework. This includes defining the goals of each experiment, ensuring randomised controlled experiments, and utilizing techniques such as one-tailed tests when appropriate. Additionally, you can benefit from complementary A/B testing methods that allow for high-tempo testing in a web experimentation context. By implementing A/B test technology and following best practices in this area, you can maximize the chances of running successful experiments that yield impactful results.
What are the essential steps to implement successful a/b testing and experimentation in my business?
To implement successful a/b testing and experimentation, you should start by defining clear objectives for your tests. Next, select common A/B tests that align with those objectives and establish a proper test design. Utilize techniques such as controlled experimentation and Barnard's test to analyze results effectively. Additionally, ensure that your experimentation program includes high-tempo testing to run multiple experiments simultaneously, thus allowing for impactful experiments that drive your strategy. Finally, maintain meticulous testing practices throughout the process to ensure valid results and insights from your experiment runs.
What techniques can I use for controlled experimentation in A/B testing when I want to initiate high-tempo testing?
For effective A/B testing and experimenting, you can employ techniques such as controlled experimentation to ensure that your tests—high-tempo testing—are reliable. To initiate your experiment start, clearly define your testing goals and utilize a structured approach to conduct the a / b testing. This will help you in refining your strategies and achieving better results in your experimentation processes.
What are some effective techniques for conducting controlled experimentation in A/B testing?
In A/B testing, controlled experimentation is a crucial technique used to ensure valid results. To successfully implement controlled experimentation, you should follow these steps: clearly define your hypotheses, randomly assign your test samples to avoid bias, and ensure that you are properly measuring the outcomes of your experiments. This method of experimentation—and the techniques utilized will allow you to make informed decisions based on reliable test data.
What techniques are used to optimize controlled experimentation in A/B testing for better results?
To optimize controlled experimentation in A/B testing, various techniques can be effectively used. These include ensuring a clear hypothesis, implementing randomization in selecting test groups, and analyzing results with proper statistical methods. By focusing on these aspects, you can enhance the quality of your experimentation and achieve more reliable outcomes.
What techniques are used to enhance controlled experimentation in A/B testing, and how do they contribute to the overall process of experimentation?
Various techniques are used to enhance controlled experimentation in A/B testing, such as stratification, randomization, and using control groups. These techniques help ensure that the results from A/B testing are valid and reliable. Additionally, controlled experimentation allows for a systematic approach, which can lead to improved outcomes and insights, ultimately benefiting the overall experimentation process.
What techniques are commonly used to test hypotheses through controlled experimentation in A/B testing?
In A/B testing, various techniques are used to optimize controlled experimentation, such as setting clear metrics, randomization, and maintaining a control group. These techniques contribute significantly to the validity of results and help improve the overall process of experimentation.
What techniques are commonly used to evaluate outcomes in controlled experimentation during A/B testing and how do they improve the overall word experimentation process?
Various techniques are used to test outcomes in controlled experimentation during A/B testing, including statistical analysis and A/B split testing methodologies, which help refine hypotheses and improve the overall word experimentation process. These techniques help ensure that the results are valid and actionable, making it easier to draw insights and make informed decisions.
What techniques are commonly used to improve the reliability of results obtained through controlled experimentation in A/B testing?
Various techniques are used to test hypotheses and ensure that controlled experimentation yields reliable outcomes. Some of the commonly used techniques in controlled experimentation involve randomized assignment, maintaining sample size, and using statistical tools to analyze the results. These techniques help to strengthen the framework of A/B testing and ensure that reliable and valid results are achieved through the experimentation process.
What techniques are commonly used to test the reliability of outcomes derived from controlled experimentation in A/B testing?
Several techniques are used to test the reliability of outcomes in controlled experimentation during A/B testing, such as statistical analysis, randomization, and power calculations. These techniques help to ensure that the results obtained from the controlled experimentation are valid and can be trusted, ultimately contributing to the overall effectiveness of A/B testing.
Related Links
What is the difference between ab testing and experimentation?What is A and B testing methodology?
What to Focus on When Setting Up A/B Tests for Google Ads