Roundup of Best Practices in A/B Testing for Ads
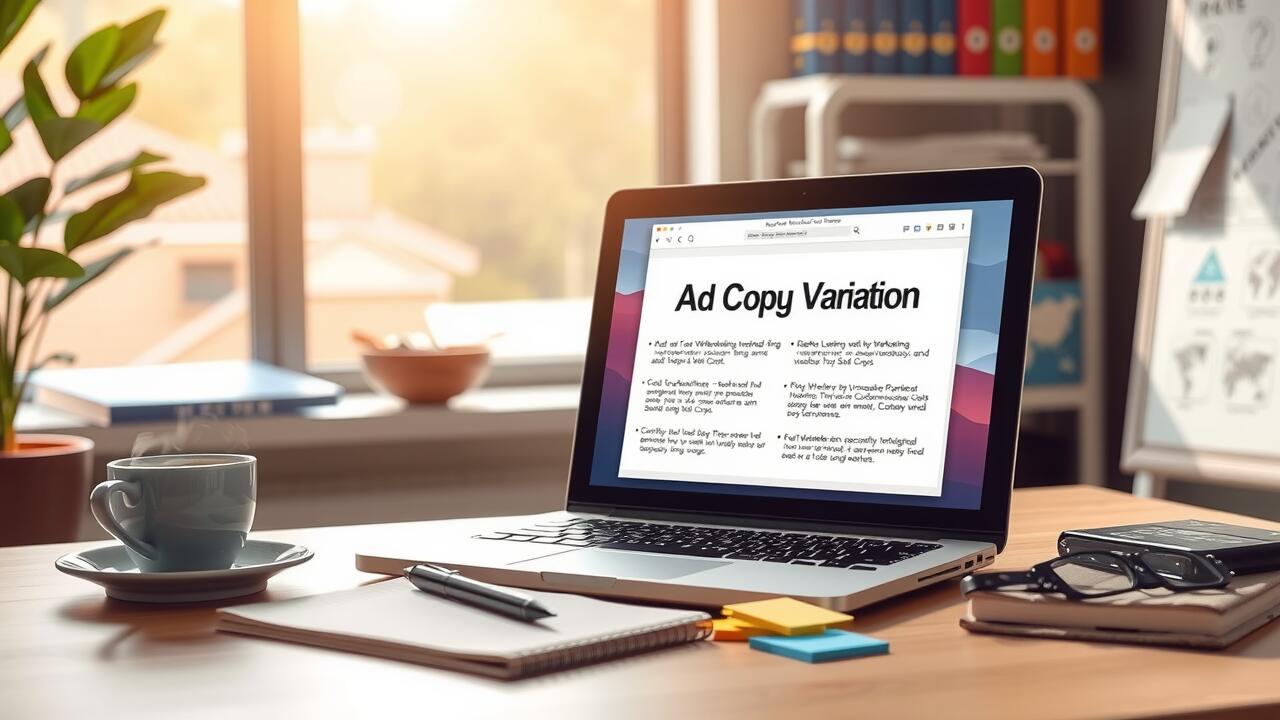
Implementing the Test
A structured approach is essential when implementing an A/B test for ads. Begin by identifying the specific element you want to test, such as headlines, images, or call-to-action buttons. Ensure that your audience is appropriately segmented to maintain the reliability of your results. Proper randomization is crucial to avoid potential biases. Each variant should be exposed to a similar number of users to ensure statistical validity.
Once you have your variants ready, consider the duration of the test. Running the test long enough to achieve significant results is vital, but it should not be overly extended, which can lead to outdated data. Monitor the testing process to confirm everything functions correctly, ensuring that traffic is equally distributed among the variants. Proper tracking and measurement tools will be necessary to collect relevant data for analysis.
Setting Up Your A/B Testing Framework
Establishing a solid framework is crucial for the success of A/B testing in advertising. Start by clearly defining your goals; understanding what you aim to achieve will guide the entire testing process. Create a hypothesis that identifies the changes you want to analyze. Select the audience segments you wish to test and ensure they are representative of your overall target market. This segmentation will help provide more relevant insights and enhance the reliability of your results.
Next, choose the elements you wish to test, such as ad copy, images, or calls to action. Keep your tests focused on a single variable to ensure that any changes in performance can be attributed directly to that variable. Determine the sample size to provide statistically significant results. A larger sample size generally leads to more reliable data, minimizing the risk of inconsistencies. Finally, decide on the duration of the test; running experiments for an appropriate length allows for variations in user behavior to be captured.
Analyzing Results
After conducting A/B tests, the next crucial step involves sifting through the collected data to glean meaningful insights. Begin by examining basic metrics such as click-through rates, conversion rates, and engagement levels. These foundational statistics provide a general overview of how each variant performed relative to the other. Ensure that the sample size is adequate to support your findings. Statistical significance should be a primary focus, as it determines whether the observed differences are likely due to chance or represent real trends in user behavior.
Delve deeper into the data to identify patterns that may not be immediately apparent. Segment your results by demographics, device types, or traffic sources, as this can reveal nuanced insights into how different audiences respond to each variant. Utilize data visualization tools to help illustrate trends and make comparisons more straightforward. Establishing correlations can lead to actionable recommendations, influencing future ad campaigns. Effective analysis should empower marketers to make data-driven decisions rather than relying solely on intuition.
Interpreting Data to Make Informed Decisions
Data interpretation is an essential step in the A/B testing process. After collecting performance metrics from the different ad variants, it is necessary to analyze these figures carefully. Focus on key performance indicators such as click-through rates, conversion rates, and overall engagement levels. Understanding which variant is performing better requires looking not just at the averages but also at variance within the data. Consider segmenting results based on demographics or behavior to gain deeper insights into how different audiences respond to each version.
Context is crucial when interpreting results. Seasonal trends or external factors can influence ad performance, skewing the data. Comparing results to historical performance can provide clarity on whether the observed changes are statistically significant. It’s also beneficial to look for patterns that emerge across different tests over time. This will help create a richer understanding of the audience’s preferences. By approaching data analysis methodically, you can make informed decisions that enhance future advertising strategies.
Drawing Conclusions
The final step in the A/B testing process involves carefully evaluating the results to determine which variant performed better. This analysis requires a clear understanding of the key performance indicators established at the beginning of the test. Minimum sample sizes must be ensured to maintain statistical significance, as drawing conclusions from insufficient data can lead to misunderstandings about campaign effectiveness.
Once the winning variant is identified, it is valuable to consider the reasons behind its success. Factors such as user engagement, conversion rates, and customer feedback can provide insights into audience behavior. This deeper understanding allows marketers to iterate on their messaging and creative approaches, facilitating continuous improvement in future advertising efforts.
Determining Winning Variants Based on Results
Identifying the winning variant in your A/B test requires a careful analysis of the data collected during the testing phase. The primary metrics to consider are conversion rates, click-through rates, and engagement metrics. Focus on statistical significance to confirm that results are not due to random chance. Tools that provide confidence intervals can aid in determining the reliability of your findings.
After evaluating the quantitative results, it’s essential to consider qualitative feedback as well. User surveys or session recordings can provide additional context for understanding why one variant outperforms another. Look for patterns in user behavior that might illuminate preferences or pain points. Combining these insights allows for more robust decision-making moving forward.
FAQS
What is A/B testing in the context of advertising?
A/B testing, or split testing, is a method used in advertising to compare two or more versions of an ad to determine which performs better based on specific metrics, such as click-through rates or conversions.
How do I set up my A/B testing framework?
To set up your A/B testing framework, define your goals, choose the variables you want to test (such as headlines or images), segment your audience, and use a reliable testing tool to randomize and control your tests.
How long should I run my A/B tests?
The duration of your A/B tests depends on your traffic and conversion rates. Generally, tests should run long enough to gather statistically significant data, which can range from a few days to several weeks.
What metrics should I focus on when analyzing A/B test results?
Key metrics to focus on include conversion rate, click-through rate, bounce rate, and return on ad spend. These will help you understand the effectiveness of each ad variant.
How do I determine which variant is the winner after an A/B test?
To determine the winner, compare the results of each variant against your defined metrics. Look for statistically significant differences that indicate one variant performed better than the others, and consider the overall impact on your campaign goals.
Related Links
A/B Testing in Ad Copy DevelopmentHow to Conduct A/B Testing for Google Ads
How to Analyse A/B Test Results Effectively