What is A and B testing methodology?
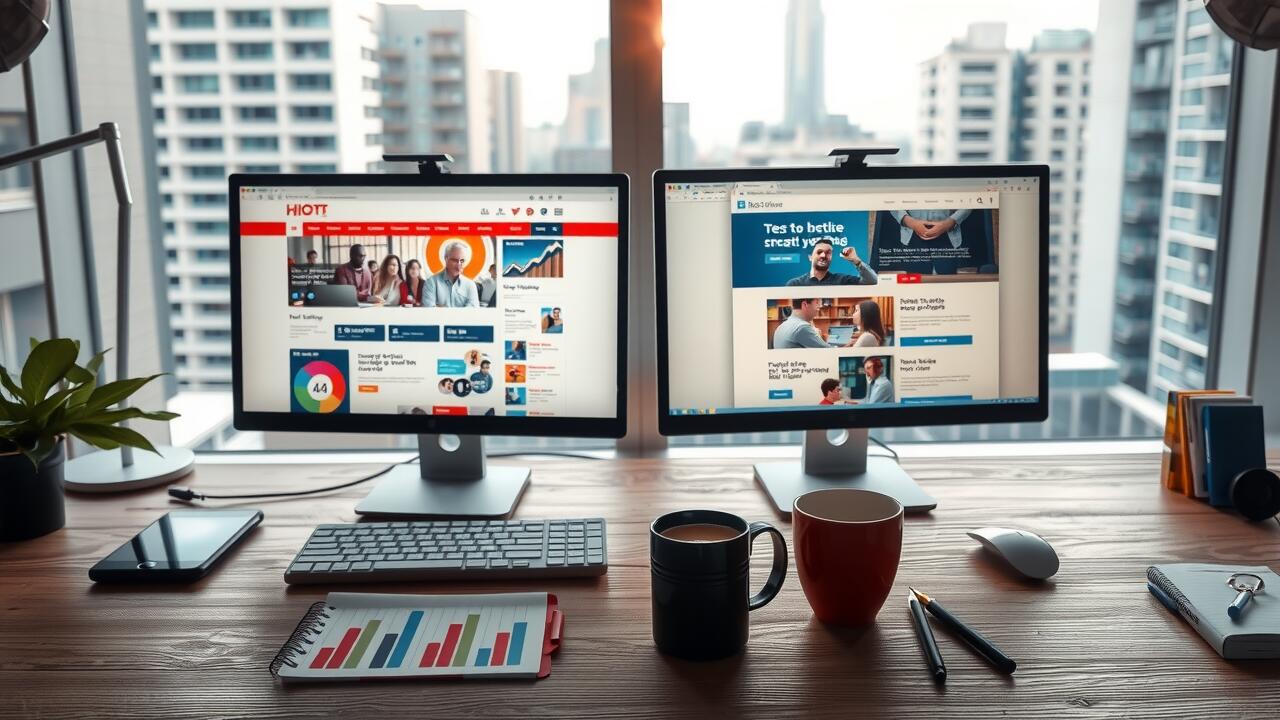
Selecting the Right Audience
Identifying an appropriate audience is crucial for any A/B testing initiative. The success of an experiment largely hinges on how well the selected audience reflects the user base or market segment that the test aims to influence. Different demographics may respond variably to changes in a product or service. Understanding behaviors and preferences of these demographics can greatly enhance the reliability of the results.
Targeting specific segments allows for more refined insights from the testing process. Factors such as age, location, and purchasing habits should be considered when selecting participants. Crafting user profiles helps in pinpointing which groups are most likely to engage with different variations of the tested element. This tailored approach not only increases the validity of the outcomes but also aids in making informed decisions for future strategies.
Targeting Demographics and Segments
Understanding the audience is crucial for effective A/B testing. By identifying specific demographics such as age, gender, location, and interests, marketers can tailor their experiments to resonate more with their target groups. For example, younger audiences may respond differently to visual content compared to older demographics. Segmenting these groups allows for a more nuanced approach, ensuring that variations tested reflect the preferences and behaviors of each audience segment.
Many tools are available to assist in analyzing these demographic details. Utilizing analytics platforms helps in gathering data to better understand user interactions and responses. This information provides insights into which segments show greater engagement with particular content types. When segments are precise, the results of A/B tests become more actionable. Ultimately, refining targeting leads to more effective marketing strategies and improved overall performance of campaigns.
Analyzing A/B Test Results
Once A/B testing concludes, the focus shifts to analyzing the collected data to derive meaningful insights. It is crucial to compare the performance of both variants using key metrics. Conversion rates, click-through rates, and engagement levels serve as primary indicators. Statistical significance should also be evaluated to determine if the observed differences are reliable or merely due to random chance. Ensuring that the sample size is adequate enhances the validity of the findings.
Data interpretation plays a critical role in determining the effectiveness of changes implemented in the test samples. Identifying patterns and trends within the results can provide deeper understanding of user behavior and preferences. Visualization tools can aid in making sense of complex data sets, making insights more accessible. Moreover, it's important to consider external factors that may have influenced user interactions. These insights form the foundation for making informed decisions in marketing strategies or product development.
Interpreting Data for Insights
Data analysis is essential in A/B testing, serving as the foundation for decision-making. Gathering metrics such as conversion rates, click-through rates, and user engagement is crucial. These figures provide insight into how each variant performs against the other. Reviewing statistical significance is also vital; it helps establish whether observed differences are meaningful or simply due to chance. Without careful analysis, the information can lead to misguided conclusions and poor business choices.
Interpreting the results involves looking beyond the numbers. Context matters in understanding why one version may outperform the other. Factors such as user feedback, behavioral trends, and market conditions play significant roles in shaping the outcome. Combining qualitative and quantitative data can reveal the underlying reasons for user preferences. This holistic approach ensures that insights gained from the tests can effectively inform future marketing strategies and product development decisions.
Common Challenges in A/B Testing
A/B testing can present several challenges that hinder effective implementation and analysis. One significant issue is the sample size. Running tests with insufficient participants can lead to unreliable results. If the audience is too small, random variations may skew data, yielding misleading insights. Additionally, improper segmentation might result in skewed outcomes. For instance, if a test does not adequately account for user behavior or demographics, the results may inaccurately reflect preferences across a broader audience.
Another challenge is the duration of the tests. Analyzing results prematurely can be tempting, especially when initial data appears promising. However, making decisions based on incomplete information can undermine the validity of the findings. Furthermore, technical issues such as tracking errors or discrepancies in data collection tools can complicate the results. Ensuring that systems are properly configured and that tracking is accurate is crucial for obtaining reliable data.
Overcoming Obstacles for Success
Navigating the complexities of A/B testing can present several challenges that may hinder the effectiveness of the methodology. One common obstacle is the difficulty in achieving statistically significant results, particularly when sample sizes are small or when traffic is limited. To address this issue, it’s essential to ensure that the sample size is adequately powered to detect differences between variations. Additionally, maintaining a clear focus on the specific goals of the test can help in designing experiments that yield meaningful insights.
Another challenge is the potential for bias in test design or interpretation of results. Researchers might inadvertently favor one variation over another due to preconceived notions or external influences. Implementing rigorous testing protocols can help mitigate such biases. Regularly reviewing the testing process and gathering input from diverse team members can also enhance objectivity. Continuous evaluation and iteration are vital for improving the testing process and achieving reliable outcomes.
FAQS
What is A/B testing?
A/B testing is a methodology used to compare two versions of a webpage, advertisement, or other content to determine which one performs better in achieving a specific goal, such as increased conversions or engagement.
How do I select the right audience for my A/B tests?
Selecting the right audience involves identifying your target demographics and segments based on factors such as age, location, interests, and behavior, ensuring that the test results are relevant and applicable to your intended users.
What metrics should I analyze during A/B testing?
Key metrics to analyze during A/B testing include conversion rates, click-through rates, engagement rates, and any other specific goals you have set for the test, which can provide insights into user behavior and preferences.
What are some common challenges faced in A/B testing?
Common challenges in A/B testing include sample size issues, external factors affecting results, reaching statistical significance, and misinterpretation of data, all of which can hinder the effectiveness of the testing process.
How can I overcome obstacles in A/B testing?
To overcome obstacles in A/B testing, ensure proper test design, use adequate sample sizes, monitor tests closely for external influences, and apply statistical analysis correctly to derive meaningful insights from the data collected.
Related Links
A/B Testing and ExperimentationWhat is the difference between ab testing and experimentation?
What to Focus on When Setting Up A/B Tests for Google Ads