What is the difference between ab testing and experimentation?
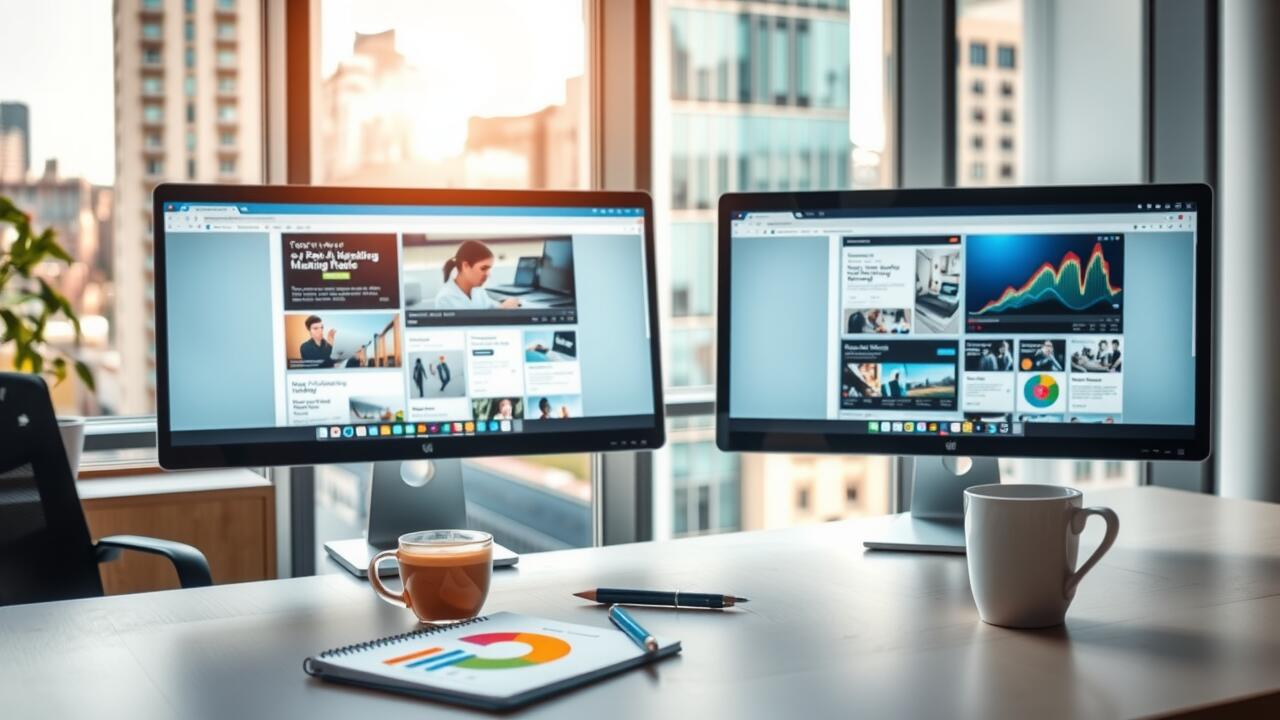
Data Analysis in A/B Testing
A/B testing focuses on comparing two versions of a variable to determine which one performs better. In this method, data analysis is centered around specific metrics like conversion rates or click-through rates. The simplicity of this approach allows for straightforward statistical comparisons, making it easier to identify winners and losers based on predetermined success criteria. This approach often employs basic statistical techniques to interpret results and establish confidence in the findings.
The data analysis process in A/B testing typically involves calculating metrics such as mean differences and variances. Researchers assess whether observed differences are statistically significant, often using a p-value to gauge the reliability of results. This emphasis on clear and concise metrics helps teams make informed decisions quickly. Despite its streamlined nature, A/B testing can provide valuable insights that guide marketing strategies, product design, and user experience improvements through focused analysis of user behavior.
Statistical Significance
In A/B testing, statistical significance plays a crucial role in determining whether the differences observed between two variations are not due to random chance. Commonly, a threshold of 0.05 p-value is used, which implies a 95% confidence level. This means that if the p-value is below this threshold, researchers can be reasonably certain that the result is likely to be genuine rather than coincidental. The implications of this are significant for making informed decisions based on test outcomes, as it relies on sound statistical principles to validate hypotheses.
Experimentation expands upon the concept of statistical significance by incorporating a broader range of metrics and testing conditions. While A/B testing often focuses on single variables, experimentation may evaluate multiple factors and their interactions simultaneously. This multifaceted approach can provide a more nuanced understanding of the data, leading to insights that are not only statistically significant but also practically meaningful. Thus, while both methodologies leverage statistical significance, the context and complexity of experimentation offer deeper insights into the underlying phenomena being studied.
Data Analysis in Experimentation
In experimentation, data analysis is a more holistic process compared to A/B testing. It involves evaluating multiple variables simultaneously, allowing for a deeper understanding of complex interactions within the data. Researchers often utilize advanced statistical methods and tools to examine how different factors influence outcomes, leading to richer insights. This comprehensive approach can reveal unexpected trends and relationships that might not emerge from simpler testing methods.
Moreover, experimentation allows for the integration of qualitative data alongside quantitative metrics. By collecting feedback through surveys or interviews, analysts can gain context about user behavior that pure A/B tests may overlook. This multi-faceted analysis enhances decision-making and drives more informed strategies, ultimately leading to more effective product or service enhancements. The ability to iterate on results based on real-world performance creates a dynamic feedback loop that is essential for innovation.
Comprehensive Metrics
In the realm of experimentation, the analysis of data encompasses a broader set of metrics compared to A/B testing. This approach examines not only conversion rates but also user engagement, retention, and overall customer satisfaction. By incorporating diverse metrics, researchers can gain deeper insights into the effectiveness of a particular change, ultimately leading to more informed decisions.
Additionally, experimentation allows for the exploration of secondary effects that might not be immediately apparent. For instance, the impact of a new feature might alter user behavior in unexpected ways. By measuring these various dimensions, organizations can understand the holistic implications of their changes, paving the way for more strategic adjustments in their offerings.
Benefits of A/B Testing
A/B testing offers a streamlined method for making data-driven decisions. By comparing two variations, organizations can quickly identify which option performs better under specific conditions. This process minimizes risks associated with changes by providing a clear winner backed by real user behavior data.
Another advantage lies in its simplicity. The design and implementation of an A/B test can be straightforward, allowing teams to execute tests without extensive resources. As a result, companies can iterate rapidly, fostering a culture of continuous improvement and innovation. This agility empowers teams to adapt strategies based on tangible results, enhancing overall performance.
Quick Insights
A/B testing provides rapid results, allowing businesses to make quick adjustments in strategies. By comparing two variants, this method reveals user preferences efficiently. The focus remains on specific metrics, facilitating straightforward interpretation and decision-making. As such, organizations can adapt their approaches based on real-time feedback, ensuring they remain responsive to customer needs.
Experimentation, on the other hand, encompasses a broader scope and often involves multiple variables. This approach delves deeper into user behavior, which can lead to comprehensive insights that inform long-term strategy. While results might take longer to analyze, they offer a richer understanding of interactions and causations. Organizations leveraging experimentation can uncover nuanced insights that A/B testing might overlook.
FAQS
What is A/B testing?
A/B testing is a method of comparing two versions of a webpage or product to determine which one performs better based on a specific metric, such as conversion rates.
How does experimentation differ from A/B testing?
Experimentation encompasses a broader range of methods and approaches beyond just comparing two variations. It may include various test designs and metrics to gather comprehensive insights about user behavior.
Why is statistical significance important in A/B testing?
Statistical significance helps determine whether the observed differences between the variants in A/B testing are due to chance or reflect a true effect, ensuring reliable conclusions from the test results.
What are some comprehensive metrics used in experimentation?
Comprehensive metrics in experimentation may include user engagement, retention rates, and overall user satisfaction, providing a more holistic view of the changes being tested.
What are the benefits of A/B testing?
A/B testing offers quick insights into user preferences, allows for data-driven decision-making, and helps optimize conversions by identifying the most effective design or content variations.
Related Links
A/B Testing and ExperimentationWhat is A and B testing methodology?
What to Focus on When Setting Up A/B Tests for Google Ads